- Nov 26, 2017
-
-
hyukjinkwon authored
## What changes were proposed in this pull request? This is a followup to reduce AppVeyor test time. This PR proposes to reduce the number of shuffle partitions to reduce the tasks running R workers in few particular tests. The symptom is similar as described in `https://github.com/apache/spark/pull/19722`. There are many R processes newly launched on Windows without forking and it makes the differences of elapsed time between Linux and Windows. Here is the simple comparison for before/after of this change. I manually tested this by disabling `spark.sparkr.use.daemon`. Disabling it resembles the tests on Windows: **Before** <img width="672" alt="2017-11-25 12 22 13" src="https://user-images.githubusercontent.com/6477701/33217949-b5528dfa-d17d-11e7-8050-75675c39eb20.png"> **After** <img width="682" alt="2017-11-25 12 32 00" src="https://user-images.githubusercontent.com/6477701/33217958-c6518052-d17d-11e7-9f8e-1be21a784559.png"> So, this probably will reduce roughly more than 10 minutes. ## How was this patch tested? AppVeyor tests Author: hyukjinkwon <gurwls223@gmail.com> Closes #19816 from HyukjinKwon/SPARK-21693-followup.
-
Sean Owen authored
## What changes were proposed in this pull request? Set `-ea` and `-Xss4m` consistently for tests, to fix in particular: ``` OrderingSuite: ... - GenerateOrdering with ShortType *** RUN ABORTED *** java.lang.StackOverflowError: at org.codehaus.janino.CodeContext.flowAnalysis(CodeContext.java:370) at org.codehaus.janino.CodeContext.flowAnalysis(CodeContext.java:541) at org.codehaus.janino.CodeContext.flowAnalysis(CodeContext.java:541) at org.codehaus.janino.CodeContext.flowAnalysis(CodeContext.java:541) at org.codehaus.janino.CodeContext.flowAnalysis(CodeContext.java:541) at org.codehaus.janino.CodeContext.flowAnalysis(CodeContext.java:541) at org.codehaus.janino.CodeContext.flowAnalysis(CodeContext.java:541) at org.codehaus.janino.CodeContext.flowAnalysis(CodeContext.java:541) ... ``` ## How was this patch tested? Existing tests. Manually verified it resolves the StackOverflowError this intends to resolve. Author: Sean Owen <sowen@cloudera.com> Closes #19820 from srowen/SPARK-22607.
-
- Nov 25, 2017
-
-
Kalvin Chau authored
The first scheduled renewal time is is set to the exact expiration time, and all subsequent renewal times are 75% of the renewal time. This makes it so that the inital renewal time is also 75%. ## What changes were proposed in this pull request? Set the initial renewal time to be 75% of renewal time. ## How was this patch tested? Tested locally in a test HDFS cluster, checking various renewal times. Author: Kalvin Chau <kalvin.chau@viasat.com> Closes #19798 from kalvinnchau/fix-inital-renewal-time.
-
Wenchen Fan authored
## What changes were proposed in this pull request? `nullsNativeAddress` and `valuesNativeAddress` are only used in tests and benchmark, no need to be top class API. ## How was this patch tested? existing tests Author: Wenchen Fan <wenchen@databricks.com> Closes #19818 from cloud-fan/minor.
-
- Nov 24, 2017
-
-
Wenchen Fan authored
## What changes were proposed in this pull request? `ctx.currentVars` means the input variables for the current operator, which is already decided in `CodegenSupport`, we can set it there instead of `doConsume`. also add more comments to help people understand the codegen framework. After this PR, we now have a principle about setting `ctx.currentVars` and `ctx.INPUT_ROW`: 1. for non-whole-stage-codegen path, never set them. (permit some special cases like generating ordering) 2. for whole-stage-codegen `produce` path, mostly we don't need to set them, but blocking operators may need to set them for expressions that produce data from data source, sort buffer, aggregate buffer, etc. 3. for whole-stage-codegen `consume` path, mostly we don't need to set them because `currentVars` is automatically set to child input variables and `INPUT_ROW` is mostly not used. A few plans need to tweak them as they may have different inputs, or they use the input row. ## How was this patch tested? existing tests. Author: Wenchen Fan <wenchen@databricks.com> Closes #19803 from cloud-fan/codegen.
-
hyukjinkwon authored
## What changes were proposed in this pull request? This PR proposes to add cmd scripts so that Windows users can also run `spark-sql` script. ## How was this patch tested? Manually tested on Windows. **Before** ```cmd C:\...\spark>.\bin\spark-sql '.\bin\spark-sql' is not recognized as an internal or external command, operable program or batch file. C:\...\spark>.\bin\spark-sql.cmd '.\bin\spark-sql.cmd' is not recognized as an internal or external command, operable program or batch file. ``` **After** ```cmd C:\...\spark>.\bin\spark-sql ... spark-sql> SELECT 'Hello World !!'; ... Hello World !! ``` Author: hyukjinkwon <gurwls223@gmail.com> Closes #19808 from HyukjinKwon/spark-sql-cmd.
-
GuoChenzhao authored
## What changes were proposed in this pull request? In adaptive execution, the map output statistics of all mappers will be aggregated after previous stage is successfully executed. Driver takes the aggregation job while it will get slow when the number of `mapper * shuffle partitions` is large, since it only uses single thread to compute. This PR uses multi-thread to deal with this single point bottleneck. ## How was this patch tested? Test cases are in `MapOutputTrackerSuite.scala` Author: GuoChenzhao <chenzhao.guo@intel.com> Author: gczsjdy <gczsjdy1994@gmail.com> Closes #19763 from gczsjdy/single_point_mapstatistics.
-
Wang Gengliang authored
## What changes were proposed in this pull request? Currently history server v2 failed to start if `listing.ldb` is corrupted. This patch get rid of the corrupted `listing.ldb` and re-create it. The exception handling follows [opening disk store for app](https://github.com/apache/spark/blob/0ffa7c488fa8156e2a1aa282e60b7c36b86d8af8/core/src/main/scala/org/apache/spark/deploy/history/FsHistoryProvider.scala#L307) ## How was this patch tested? manual test Please review http://spark.apache.org/contributing.html before opening a pull request. Author: Wang Gengliang <ltnwgl@gmail.com> Closes #19786 from gengliangwang/listingException.
-
Kazuaki Ishizaki authored
[SPARK-22595][SQL] fix flaky test: CastSuite.SPARK-22500: cast for struct should not generate codes beyond 64KB ## What changes were proposed in this pull request? This PR reduces the number of fields in the test case of `CastSuite` to fix an issue that is pointed at [here](https://github.com/apache/spark/pull/19800#issuecomment-346634950). ``` java.lang.OutOfMemoryError: GC overhead limit exceeded java.lang.OutOfMemoryError: GC overhead limit exceeded at org.codehaus.janino.UnitCompiler.findClass(UnitCompiler.java:10971) at org.codehaus.janino.UnitCompiler.findTypeByName(UnitCompiler.java:7607) at org.codehaus.janino.UnitCompiler.getReferenceType(UnitCompiler.java:5758) at org.codehaus.janino.UnitCompiler.getType2(UnitCompiler.java:5732) at org.codehaus.janino.UnitCompiler.access$13200(UnitCompiler.java:206) at org.codehaus.janino.UnitCompiler$18.visitReferenceType(UnitCompiler.java:5668) at org.codehaus.janino.UnitCompiler$18.visitReferenceType(UnitCompiler.java:5660) at org.codehaus.janino.Java$ReferenceType.accept(Java.java:3356) at org.codehaus.janino.UnitCompiler.getType(UnitCompiler.java:5660) at org.codehaus.janino.UnitCompiler.buildLocalVariableMap(UnitCompiler.java:2892) at org.codehaus.janino.UnitCompiler.compile(UnitCompiler.java:2764) at org.codehaus.janino.UnitCompiler.compileDeclaredMethods(UnitCompiler.java:1262) at org.codehaus.janino.UnitCompiler.compileDeclaredMethods(UnitCompiler.java:1234) at org.codehaus.janino.UnitCompiler.compile2(UnitCompiler.java:538) at org.codehaus.janino.UnitCompiler.compile2(UnitCompiler.java:890) at org.codehaus.janino.UnitCompiler.compile2(UnitCompiler.java:894) at org.codehaus.janino.UnitCompiler.access$600(UnitCompiler.java:206) at org.codehaus.janino.UnitCompiler$2.visitMemberClassDeclaration(UnitCompiler.java:377) at org.codehaus.janino.UnitCompiler$2.visitMemberClassDeclaration(UnitCompiler.java:369) at org.codehaus.janino.Java$MemberClassDeclaration.accept(Java.java:1128) at org.codehaus.janino.UnitCompiler.compile(UnitCompiler.java:369) at org.codehaus.janino.UnitCompiler.compileDeclaredMemberTypes(UnitCompiler.java:1209) at org.codehaus.janino.UnitCompiler.compile2(UnitCompiler.java:564) at org.codehaus.janino.UnitCompiler.compile2(UnitCompiler.java:890) at org.codehaus.janino.UnitCompiler.compile2(UnitCompiler.java:894) at org.codehaus.janino.UnitCompiler.access$600(UnitCompiler.java:206) at org.codehaus.janino.UnitCompiler$2.visitMemberClassDeclaration(UnitCompiler.java:377) at org.codehaus.janino.UnitCompiler$2.visitMemberClassDeclaration(UnitCompiler.java:369) at org.codehaus.janino.Java$MemberClassDeclaration.accept(Java.java:1128) at org.codehaus.janino.UnitCompiler.compile(UnitCompiler.java:369) at org.codehaus.janino.UnitCompiler.compileDeclaredMemberTypes(UnitCompiler.java:1209) at org.codehaus.janino.UnitCompiler.compile2(UnitCompiler.java:564) ... ``` ## How was this patch tested? Used existing test case Author: Kazuaki Ishizaki <ishizaki@jp.ibm.com> Closes #19806 from kiszk/SPARK-22595.
-
Liang-Chi Hsieh authored
## What changes were proposed in this pull request? When I played with codegen in developing another PR, I found the value of `CodegenContext.INPUT_ROW` is not reliable. Under wholestage codegen, it is assigned to null first and then suddenly changed to `i`. The reason is `GenerateOrdering` changes `CodegenContext.INPUT_ROW` but doesn't restore it back. ## How was this patch tested? Added test. Author: Liang-Chi Hsieh <viirya@gmail.com> Closes #19800 from viirya/SPARK-22591.
-
- Nov 23, 2017
-
-
Wenchen Fan authored
## What changes were proposed in this pull request? We have 2 different methods to convert filters for hive, regarding a config. This introduces duplicated and inconsistent code(e.g. one use helper objects for pattern match and one doesn't). ## How was this patch tested? existing tests Author: Wenchen Fan <wenchen@databricks.com> Closes #19801 from cloud-fan/cleanup.
-
Wenchen Fan authored
## What changes were proposed in this pull request? a followup of https://github.com/apache/spark/pull/19779 , to simplify the file creation. ## How was this patch tested? test only change Author: Wenchen Fan <wenchen@databricks.com> Closes #19799 from cloud-fan/minor.
-
- Nov 22, 2017
-
-
Jakub Nowacki authored
## What changes were proposed in this pull request? Fixing the way how `SPARK_HOME` is resolved on Windows. While the previous version was working with the built release download, the set of directories changed slightly for the PySpark `pip` or `conda` install. This has been reflected in Linux files in `bin` but not for Windows `cmd` files. First fix improves the way how the `jars` directory is found, as this was stoping Windows version of `pip/conda` install from working; JARs were not found by on Session/Context setup. Second fix is adding `find-spark-home.cmd` script, which uses `find_spark_home.py` script, as the Linux version, to resolve `SPARK_HOME`. It is based on `find-spark-home` bash script, though, some operations are done in different order due to the `cmd` script language limitations. If environment variable is set, the Python script `find_spark_home.py` will not be run. The process can fail if Python is not installed, but it will mostly use this way if PySpark is installed via `pip/conda`, thus, there is some Python in the system. ## How was this patch tested? Tested on local installation. Author: Jakub Nowacki <j.s.nowacki@gmail.com> Closes #19370 from jsnowacki/fix_spark_cmds.
-
Ilya Matiach authored
## What changes were proposed in this pull request? Adding spark image reader, an implementation of schema for representing images in spark DataFrames The code is taken from the spark package located here: (https://github.com/Microsoft/spark-images) Please see the JIRA for more information (https://issues.apache.org/jira/browse/SPARK-21866) Please see mailing list for SPIP vote and approval information: (http://apache-spark-developers-list.1001551.n3.nabble.com/VOTE-SPIP-SPARK-21866-Image-support-in-Apache-Spark-td22510.html) # Background and motivation As Apache Spark is being used more and more in the industry, some new use cases are emerging for different data formats beyond the traditional SQL types or the numerical types (vectors and matrices). Deep Learning applications commonly deal with image processing. A number of projects add some Deep Learning capabilities to Spark (see list below), but they struggle to communicate with each other or with MLlib pipelines because there is no standard way to represent an image in Spark DataFrames. We propose to federate efforts for representing images in Spark by defining a representation that caters to the most common needs of users and library developers. This SPIP proposes a specification to represent images in Spark DataFrames and Datasets (based on existing industrial standards), and an interface for loading sources of images. It is not meant to be a full-fledged image processing library, but rather the core description that other libraries and users can rely on. Several packages already offer various processing facilities for transforming images or doing more complex operations, and each has various design tradeoffs that make them better as standalone solutions. This project is a joint collaboration between Microsoft and Databricks, which have been testing this design in two open source packages: MMLSpark and Deep Learning Pipelines. The proposed image format is an in-memory, decompressed representation that targets low-level applications. It is significantly more liberal in memory usage than compressed image representations such as JPEG, PNG, etc., but it allows easy communication with popular image processing libraries and has no decoding overhead. ## How was this patch tested? Unit tests in scala ImageSchemaSuite, unit tests in python Author: Ilya Matiach <ilmat@microsoft.com> Author: hyukjinkwon <gurwls223@gmail.com> Closes #19439 from imatiach-msft/ilmat/spark-images.
-
Wenchen Fan authored
## What changes were proposed in this pull request? A frequently reported issue of Spark is the Java 64kb compile error. This is because Spark generates a very big method and it's usually caused by 3 reasons: 1. a deep expression tree, e.g. a very complex filter condition 2. many individual expressions, e.g. expressions can have many children, operators can have many expressions. 3. a deep query plan tree (with whole stage codegen) This PR focuses on 1. There are already several patches(#15620 #18972 #18641) trying to fix this issue and some of them are already merged. However this is an endless job as every non-leaf expression has this issue. This PR proposes to fix this issue in `Expression.genCode`, to make sure the code for a single expression won't grow too big. According to maropu 's benchmark, no regression is found with TPCDS (thanks maropu !): https://docs.google.com/spreadsheets/d/1K3_7lX05-ZgxDXi9X_GleNnDjcnJIfoSlSCDZcL4gdg/edit?usp=sharing ## How was this patch tested? existing test Author: Wenchen Fan <wenchen@databricks.com> Author: Wenchen Fan <cloud0fan@gmail.com> Closes #19767 from cloud-fan/codegen.
-
Mark Petruska authored
## What changes were proposed in this pull request? Ticket: [SPARK-22572](https://issues.apache.org/jira/browse/SPARK-22572) ## How was this patch tested? Added a new test case to `org.apache.spark.repl.ReplSuite` Author: Mark Petruska <petruska.mark@gmail.com> Closes #19791 from mpetruska/SPARK-22572.
-
Kazuaki Ishizaki authored
## What changes were proposed in this pull request? This PR addresses [the spelling miss](https://github.com/apache/spark/pull/17436#discussion_r152189670) of the config name `spark.sql.columnVector.offheap.enabled`. We should use `spark.sql.columnVector.offheap.enabled`. ## How was this patch tested? Existing tests Author: Kazuaki Ishizaki <ishizaki@jp.ibm.com> Closes #19794 from kiszk/SPARK-20101-follow.
-
Takeshi Yamamuro authored
## What changes were proposed in this pull request? I found #19656 causes some bugs, for example, it changed the result set of `q6` in tpcds (I keep tracking TPCDS results daily [here](https://github.com/maropu/spark-tpcds-datagen/tree/master/reports/tests)): - w/o pr19658 ``` +-----+---+ |state|cnt| +-----+---+ | MA| 10| | AK| 10| | AZ| 11| | ME| 13| | VT| 14| | NV| 15| | NH| 16| | UT| 17| | NJ| 21| | MD| 22| | WY| 25| | NM| 26| | OR| 31| | WA| 36| | ND| 38| | ID| 39| | SC| 45| | WV| 50| | FL| 51| | OK| 53| | MT| 53| | CO| 57| | AR| 58| | NY| 58| | PA| 62| | AL| 63| | LA| 63| | SD| 70| | WI| 80| | null| 81| | MI| 82| | NC| 82| | MS| 83| | CA| 84| | MN| 85| | MO| 88| | IL| 95| | IA|102| | TN|102| | IN|103| | KY|104| | NE|113| | OH|114| | VA|130| | KS|139| | GA|168| | TX|216| +-----+---+ ``` - w/ pr19658 ``` +-----+---+ |state|cnt| +-----+---+ | RI| 14| | AK| 16| | FL| 20| | NJ| 21| | NM| 21| | NV| 22| | MA| 22| | MD| 22| | UT| 22| | AZ| 25| | SC| 28| | AL| 36| | MT| 36| | WA| 39| | ND| 41| | MI| 44| | AR| 45| | OR| 47| | OK| 52| | PA| 53| | LA| 55| | CO| 55| | NY| 64| | WV| 66| | SD| 72| | MS| 73| | NC| 79| | IN| 82| | null| 85| | ID| 88| | MN| 91| | WI| 95| | IL| 96| | MO| 97| | CA|109| | CA|109| | TN|114| | NE|115| | KY|128| | OH|131| | IA|156| | TX|160| | VA|182| | KS|211| | GA|230| +-----+---+ ``` This pr is to keep the original logic of `CodegenContext.copyResult` in `BroadcastHashJoinExec`. ## How was this patch tested? Existing tests Author: Takeshi Yamamuro <yamamuro@apache.org> Closes #19781 from maropu/SPARK-22445-bugfix.
-
vinodkc authored
[SPARK-17920][SPARK-19580][SPARK-19878][SQL] Support writing to Hive table which uses Avro schema url 'avro.schema.url' ## What changes were proposed in this pull request? SPARK-19580 Support for avro.schema.url while writing to hive table SPARK-19878 Add hive configuration when initialize hive serde in InsertIntoHiveTable.scala SPARK-17920 HiveWriterContainer passes null configuration to serde.initialize, causing NullPointerException in AvroSerde when using avro.schema.url Support writing to Hive table which uses Avro schema url 'avro.schema.url' For ex: create external table avro_in (a string) stored as avro location '/avro-in/' tblproperties ('avro.schema.url'='/avro-schema/avro.avsc'); create external table avro_out (a string) stored as avro location '/avro-out/' tblproperties ('avro.schema.url'='/avro-schema/avro.avsc'); insert overwrite table avro_out select * from avro_in; // fails with java.lang.NullPointerException WARN AvroSerDe: Encountered exception determining schema. Returning signal schema to indicate problem java.lang.NullPointerException at org.apache.hadoop.fs.FileSystem.getDefaultUri(FileSystem.java:182) at org.apache.hadoop.fs.FileSystem.get(FileSystem.java:174) ## Changes proposed in this fix Currently 'null' value is passed to serializer, which causes NPE during insert operation, instead pass Hadoop configuration object ## How was this patch tested? Added new test case in VersionsSuite Author: vinodkc <vinod.kc.in@gmail.com> Closes #19779 from vinodkc/br_Fix_SPARK-17920.
-
- Nov 21, 2017
-
-
Jia Li authored
## What changes were proposed in this pull request? Let’s say I have a nested AND expression shown below and p2 can not be pushed down, (p1 AND p2) OR p3 In current Spark code, during data source filter translation, (p1 AND p2) is returned as p1 only and p2 is simply lost. This issue occurs with JDBC data source and is similar to [SPARK-12218](https://github.com/apache/spark/pull/10362) for Parquet. When we have AND nested below another expression, we should either push both legs or nothing. Note that: - The current Spark code will always split conjunctive predicate before it determines if a predicate can be pushed down or not - If I have (p1 AND p2) AND p3, it will be split into p1, p2, p3. There won't be nested AND expression. - The current Spark code logic for OR is OK. It either pushes both legs or nothing. The same translation method is also called by Data Source V2. ## How was this patch tested? Added new unit test cases to JDBCSuite gatorsmile Author: Jia Li <jiali@us.ibm.com> Closes #19776 from jliwork/spark-22548.
-
Kazuaki Ishizaki authored
## What changes were proposed in this pull request? This PR changes `cast` code generation to place generated code for expression for fields of a structure into separated methods if these size could be large. ## How was this patch tested? Added new test cases into `CastSuite` Author: Kazuaki Ishizaki <ishizaki@jp.ibm.com> Closes #19730 from kiszk/SPARK-22500.
-
Marco Gaido authored
## What changes were proposed in this pull request? Added the histogram representation to the output of the `DESCRIBE EXTENDED table_name column_name` command. ## How was this patch tested? Modified SQL UT and checked output Please review http://spark.apache.org/contributing.html before opening a pull request. Author: Marco Gaido <mgaido@hortonworks.com> Closes #19774 from mgaido91/SPARK-22475.
-
hyukjinkwon authored
[SPARK-22165][SQL] Fixes type conflicts between double, long, decimals, dates and timestamps in partition column ## What changes were proposed in this pull request? This PR proposes to add a rule that re-uses `TypeCoercion.findWiderCommonType` when resolving type conflicts in partition values. Currently, this uses numeric precedence-like comparison; therefore, it looks introducing failures for type conflicts between timestamps, dates and decimals, please see: ```scala private val upCastingOrder: Seq[DataType] = Seq(NullType, IntegerType, LongType, FloatType, DoubleType, StringType) ... literals.map(_.dataType).maxBy(upCastingOrder.indexOf(_)) ``` The codes below: ```scala val df = Seq((1, "2015-01-01"), (2, "2016-01-01 00:00:00")).toDF("i", "ts") df.write.format("parquet").partitionBy("ts").save("/tmp/foo") spark.read.load("/tmp/foo").printSchema() val df = Seq((1, "1"), (2, "1" * 30)).toDF("i", "decimal") df.write.format("parquet").partitionBy("decimal").save("/tmp/bar") spark.read.load("/tmp/bar").printSchema() ``` produces output as below: **Before** ``` root |-- i: integer (nullable = true) |-- ts: date (nullable = true) root |-- i: integer (nullable = true) |-- decimal: integer (nullable = true) ``` **After** ``` root |-- i: integer (nullable = true) |-- ts: timestamp (nullable = true) root |-- i: integer (nullable = true) |-- decimal: decimal(30,0) (nullable = true) ``` ### Type coercion table: This PR proposes the type conflict resolusion as below: **Before** |InputA \ InputB|`NullType`|`IntegerType`|`LongType`|`DecimalType(38,0)`|`DoubleType`|`DateType`|`TimestampType`|`StringType`| |------------------------|----------|----------|----------|----------|----------|----------|----------|----------| |**`NullType`**|`StringType`|`IntegerType`|`LongType`|`StringType`|`DoubleType`|`StringType`|`StringType`|`StringType`| |**`IntegerType`**|`IntegerType`|`IntegerType`|`LongType`|`IntegerType`|`DoubleType`|`IntegerType`|`IntegerType`|`StringType`| |**`LongType`**|`LongType`|`LongType`|`LongType`|`LongType`|`DoubleType`|`LongType`|`LongType`|`StringType`| |**`DecimalType(38,0)`**|`StringType`|`IntegerType`|`LongType`|`DecimalType(38,0)`|`DoubleType`|`DecimalType(38,0)`|`DecimalType(38,0)`|`StringType`| |**`DoubleType`**|`DoubleType`|`DoubleType`|`DoubleType`|`DoubleType`|`DoubleType`|`DoubleType`|`DoubleType`|`StringType`| |**`DateType`**|`StringType`|`IntegerType`|`LongType`|`DateType`|`DoubleType`|`DateType`|`DateType`|`StringType`| |**`TimestampType`**|`StringType`|`IntegerType`|`LongType`|`TimestampType`|`DoubleType`|`TimestampType`|`TimestampType`|`StringType`| |**`StringType`**|`StringType`|`StringType`|`StringType`|`StringType`|`StringType`|`StringType`|`StringType`|`StringType`| **After** |InputA \ InputB|`NullType`|`IntegerType`|`LongType`|`DecimalType(38,0)`|`DoubleType`|`DateType`|`TimestampType`|`StringType`| |------------------------|----------|----------|----------|----------|----------|----------|----------|----------| |**`NullType`**|`NullType`|`IntegerType`|`LongType`|`DecimalType(38,0)`|`DoubleType`|`DateType`|`TimestampType`|`StringType`| |**`IntegerType`**|`IntegerType`|`IntegerType`|`LongType`|`DecimalType(38,0)`|`DoubleType`|`StringType`|`StringType`|`StringType`| |**`LongType`**|`LongType`|`LongType`|`LongType`|`DecimalType(38,0)`|`StringType`|`StringType`|`StringType`|`StringType`| |**`DecimalType(38,0)`**|`DecimalType(38,0)`|`DecimalType(38,0)`|`DecimalType(38,0)`|`DecimalType(38,0)`|`StringType`|`StringType`|`StringType`|`StringType`| |**`DoubleType`**|`DoubleType`|`DoubleType`|`StringType`|`StringType`|`DoubleType`|`StringType`|`StringType`|`StringType`| |**`DateType`**|`DateType`|`StringType`|`StringType`|`StringType`|`StringType`|`DateType`|`TimestampType`|`StringType`| |**`TimestampType`**|`TimestampType`|`StringType`|`StringType`|`StringType`|`StringType`|`TimestampType`|`TimestampType`|`StringType`| |**`StringType`**|`StringType`|`StringType`|`StringType`|`StringType`|`StringType`|`StringType`|`StringType`|`StringType`| This was produced by: ```scala test("Print out chart") { val supportedTypes: Seq[DataType] = Seq( NullType, IntegerType, LongType, DecimalType(38, 0), DoubleType, DateType, TimestampType, StringType) // Old type conflict resolution: val upCastingOrder: Seq[DataType] = Seq(NullType, IntegerType, LongType, FloatType, DoubleType, StringType) def oldResolveTypeConflicts(dataTypes: Seq[DataType]): DataType = { val topType = dataTypes.maxBy(upCastingOrder.indexOf(_)) if (topType == NullType) StringType else topType } println(s"|InputA \\ InputB|${supportedTypes.map(dt => s"`${dt.toString}`").mkString("|")}|") println(s"|------------------------|${supportedTypes.map(_ => "----------").mkString("|")}|") supportedTypes.foreach { inputA => val types = supportedTypes.map(inputB => oldResolveTypeConflicts(Seq(inputA, inputB))) println(s"|**`$inputA`**|${types.map(dt => s"`${dt.toString}`").mkString("|")}|") } // New type conflict resolution: def newResolveTypeConflicts(dataTypes: Seq[DataType]): DataType = { dataTypes.fold[DataType](NullType)(findWiderTypeForPartitionColumn) } println(s"|InputA \\ InputB|${supportedTypes.map(dt => s"`${dt.toString}`").mkString("|")}|") println(s"|------------------------|${supportedTypes.map(_ => "----------").mkString("|")}|") supportedTypes.foreach { inputA => val types = supportedTypes.map(inputB => newResolveTypeConflicts(Seq(inputA, inputB))) println(s"|**`$inputA`**|${types.map(dt => s"`${dt.toString}`").mkString("|")}|") } } ``` ## How was this patch tested? Unit tests added in `ParquetPartitionDiscoverySuite`. Author: hyukjinkwon <gurwls223@gmail.com> Closes #19389 from HyukjinKwon/partition-type-coercion.
-
WeichenXu authored
## What changes were proposed in this pull request? Add python api for VectorIndexerModel support handle unseen categories via handleInvalid. ## How was this patch tested? doctest added. Author: WeichenXu <weichen.xu@databricks.com> Closes #19753 from WeichenXu123/vector_indexer_invalid_py.
-
Prashant Sharma authored
## What changes were proposed in this pull request? A minor CSS style change to make Left navigation bar stay fixed with respect to scrolling, it improves usability of the docs. ## How was this patch tested? It was tested on both, firefox and chrome. ### Before 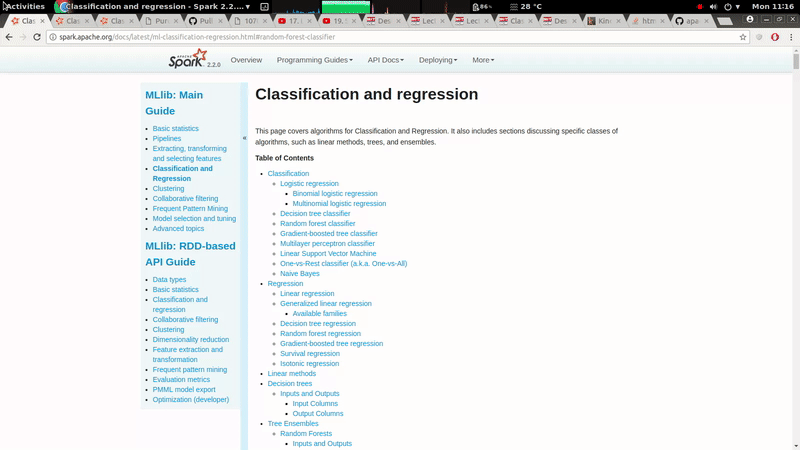 ### After 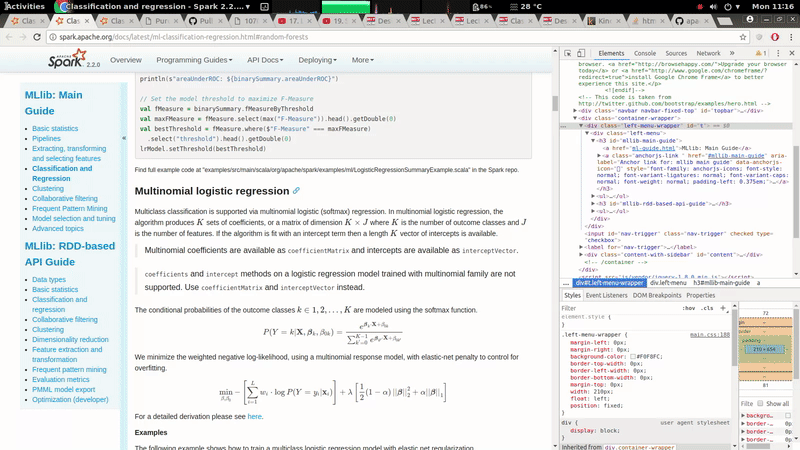 Author: Prashant Sharma <prashsh1@in.ibm.com> Closes #19785 from ScrapCodes/doc/css.
-
gatorsmile authored
## What changes were proposed in this pull request? This PR is to clean the usage of addMutableState and splitExpressions 1. replace hardcoded type string to ctx.JAVA_BOOLEAN etc. 2. create a default value of the initCode for ctx.addMutableStats 3. Use named arguments when calling `splitExpressions ` ## How was this patch tested? The existing test cases Author: gatorsmile <gatorsmile@gmail.com> Closes #19790 from gatorsmile/codeClean.
-
Kazuaki Ishizaki authored
## What changes were proposed in this pull request? This PR changes `elt` code generation to place generated code for expression for arguments into separated methods if these size could be large. This PR resolved the case of `elt` with a lot of argument ## How was this patch tested? Added new test cases into `StringExpressionsSuite` Author: Kazuaki Ishizaki <ishizaki@jp.ibm.com> Closes #19778 from kiszk/SPARK-22550.
-
Kazuaki Ishizaki authored
## What changes were proposed in this pull request? This PR changes `GenerateUnsafeRowJoiner.create()` code generation to place generated code for statements to operate bitmap and offset into separated methods if these size could be large. ## How was this patch tested? Added a new test case into `GenerateUnsafeRowJoinerSuite` Author: Kazuaki Ishizaki <ishizaki@jp.ibm.com> Closes #19737 from kiszk/SPARK-22508.
-
Liang-Chi Hsieh authored
[SPARK-22541][SQL] Explicitly claim that Python udfs can't be conditionally executed with short-curcuit evaluation ## What changes were proposed in this pull request? Besides conditional expressions such as `when` and `if`, users may want to conditionally execute python udfs by short-curcuit evaluation. We should also explicitly note that python udfs don't support this kind of conditional execution too. ## How was this patch tested? N/A, just document change. Author: Liang-Chi Hsieh <viirya@gmail.com> Closes #19787 from viirya/SPARK-22541.
-
- Nov 20, 2017
-
-
Kazuaki Ishizaki authored
## What changes were proposed in this pull request? This PR changes `concat_ws` code generation to place generated code for expression for arguments into separated methods if these size could be large. This PR resolved the case of `concat_ws` with a lot of argument ## How was this patch tested? Added new test cases into `StringExpressionsSuite` Author: Kazuaki Ishizaki <ishizaki@jp.ibm.com> Closes #19777 from kiszk/SPARK-22549.
-
Marcelo Vanzin authored
This change hooks up the config reader to `SparkConf.getDeprecatedConfig`, so that config constants with deprecated names generate the proper warnings. It also changes two deprecated configs from the new "alternatives" system to the old deprecation system, since they're not yet hooked up to each other. Added a few unit tests to verify the desired behavior. Author: Marcelo Vanzin <vanzin@cloudera.com> Closes #19760 from vanzin/SPARK-22533.
-
Kazuaki Ishizaki authored
[SPARK-20101][SQL] Use OffHeapColumnVector when "spark.sql.columnVector.offheap.enable" is set to "true" This PR enables to use ``OffHeapColumnVector`` when ``spark.sql.columnVector.offheap.enable`` is set to ``true``. While ``ColumnVector`` has two implementations ``OnHeapColumnVector`` and ``OffHeapColumnVector``, only ``OnHeapColumnVector`` is always used. This PR implements the followings - Pass ``OffHeapColumnVector`` to ``ColumnarBatch.allocate()`` when ``spark.sql.columnVector.offheap.enable`` is set to ``true`` - Free all of off-heap memory regions by ``OffHeapColumnVector.close()`` - Ensure to call ``OffHeapColumnVector.close()`` Use existing tests Author: Kazuaki Ishizaki <ishizaki@jp.ibm.com> Closes #17436 from kiszk/SPARK-20101.
-
- Nov 19, 2017
-
-
hyukjinkwon authored
## What changes were proposed in this pull request? This PR proposes to add a flag to control if PySpark should use daemon or not. Actually, SparkR already has a flag for useDaemon: https://github.com/apache/spark/blob/478fbc866fbfdb4439788583281863ecea14e8af/core/src/main/scala/org/apache/spark/api/r/RRunner.scala#L362 It'd be great if we have this flag too. It makes easier to debug Windows specific issue. ## How was this patch tested? Manually tested. Author: hyukjinkwon <gurwls223@gmail.com> Closes #19782 from HyukjinKwon/use-daemon-flag.
-
Dongjoon Hyun authored
## What changes were proposed in this pull request? ScalaTest 3.0 uses an implicit `Signaler`. This PR makes it sure all Spark tests uses `ThreadSignaler` explicitly which has the same default behavior of interrupting a thread on the JVM like ScalaTest 2.2.x. This will reduce potential flakiness. ## How was this patch tested? This is testsuite-only update. This should passes the Jenkins tests. Author: Dongjoon Hyun <dongjoon@apache.org> Closes #19784 from dongjoon-hyun/use_thread_signaler.
-
- Nov 18, 2017
-
-
Kazuaki Ishizaki authored
## What changes were proposed in this pull request? This PR changes `concat` code generation to place generated code for expression for arguments into separated methods if these size could be large. This PR resolved the case of `concat` with a lot of argument ## How was this patch tested? Added new test cases into `StringExpressionsSuite` Author: Kazuaki Ishizaki <ishizaki@jp.ibm.com> Closes #19728 from kiszk/SPARK-22498.
-
- Nov 17, 2017
-
-
Shixiong Zhu authored
## What changes were proposed in this pull request? Pass the FileSystem created using the correct Hadoop conf into `globPathIfNecessary` so that it can pick up user's hadoop configurations, such as credentials. ## How was this patch tested? Jenkins Author: Shixiong Zhu <zsxwing@gmail.com> Closes #19771 from zsxwing/fix-file-stream-conf.
-
Liang-Chi Hsieh authored
## What changes were proposed in this pull request? `SQLTransformer.transform` unpersists input dataset when dropping temporary view. We should not change input dataset's cache status. ## How was this patch tested? Added test. Author: Liang-Chi Hsieh <viirya@gmail.com> Closes #19772 from viirya/SPARK-22538.
-
Li Jin authored
## What changes were proposed in this pull request? * Add a "function type" argument to pandas_udf. * Add a new public enum class `PandasUdfType` in pyspark.sql.functions * Refactor udf related code from pyspark.sql.functions to pyspark.sql.udf * Merge "PythonUdfType" and "PythonEvalType" into a single enum class "PythonEvalType" Example: ``` from pyspark.sql.functions import pandas_udf, PandasUDFType pandas_udf('double', PandasUDFType.SCALAR): def plus_one(v): return v + 1 ``` ## Design doc https://docs.google.com/document/d/1KlLaa-xJ3oz28xlEJqXyCAHU3dwFYkFs_ixcUXrJNTc/edit ## How was this patch tested? Added PandasUDFTests ## TODO: * [x] Implement proper enum type for `PandasUDFType` * [x] Update documentation * [x] Add more tests in PandasUDFTests Author: Li Jin <ice.xelloss@gmail.com> Closes #19630 from icexelloss/spark-22409-pandas-udf-type.
-
yucai authored
## What changes were proposed in this pull request? Ensure HighlyCompressedMapStatus calculates correct avgSize ## How was this patch tested? New unit test added. Author: yucai <yucai.yu@intel.com> Closes #19765 from yucai/avgsize.
-
- Nov 16, 2017
-
-
Wenchen Fan authored
## What changes were proposed in this pull request? `ColumnarBatch` provides features to do fast filter and project in a columnar fashion, however this feature is never used by Spark, as Spark uses whole stage codegen and processes the data in a row fashion. This PR proposes to remove these unused features as we won't switch to columnar execution in the near future. Even we do, I think this part needs a proper redesign. This is also a step to make `ColumnVector` public, as we don't wanna expose these features to users. ## How was this patch tested? existing tests Author: Wenchen Fan <wenchen@databricks.com> Closes #19766 from cloud-fan/vector.
-